What Happens When HVAC and AI Meet Over Drinks
HVAC: Bro, I heard you’re new in the town. I have been living here since 1902 when my great-great-great grandfather Willis Carrier came to settle here.
AI: Yes, I heard about your deep roots. Actually, I am relatively new, just 9-10 years living here. But only recently, people have started noticing me.
HVAC: I have heard a lot about you, especially how “intelligent” you are and so on. But what exactly do you do?
AI: Well, my intelligence is based on a unique ability of mine: I can learn, just like humans learn. For example, I can learn to drive cars, diagnose diseases, and predict housing prices.
HVAC: That’s great! But what kind of study materials do you need to learn?
AI: Bro, I need only one thing: data. I can drink insane amounts of data and learn from it, figuring out patterns and relationships between multiple variables.
HVAC: I see! You drink data, and I drink energy. Ha! Ha! Ha!
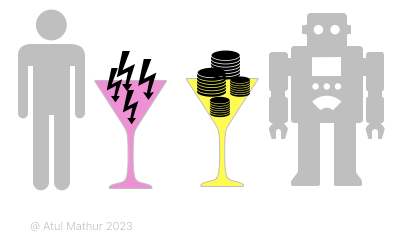
AI: Alright, let me give you a sense of my bread-and-butter capabilities. I am good at regression, classification, and clustering. For the moment, I am leaving aside the generative AI capabilities that everyone is talking about.
HVAC: Bro, I am a simple old guy; I can’t understand these terms. Please explain to me with examples.
AI: No worries, let me share a few examples.
Regression
AI: Basically, regression is nothing but figuring out complicated relationships between variables to predict something.
Let’s say you’ve got a dataset of 10,000 people, comprising their age, level in the corporate hierarchy, BMI scores, sleeping hours, whether they exercise or not, time spent in meetings, time spent on social media, commuting time, meditation/yoga or not, etc. And also their stress levels on a scale of 1 to 10.
If you give me this data, I can determine the relationship between all those data points (age, sleeping, BMI, etc.) and their stress level. Once I do it, I am ready with what is known as “model.”
HVAC: Okay, but what do I do with that model?
AI: You can simply feed the features of any new person into the model—and it can quite reliably predict their stress level. Think about it: You visit a doctor, and she asks a few questions—and then feeds the data into the model and can give you not just medicines but also some advice on reducing stress. Isn’t it life-saving?
HVAC: That’s cool, man! I won’t ask how you do it, but it looks amazing.
AI: In a similar way, people use me for predicting stock prices, economic growth, agriculture yield, and so on. If I get a dataset comprising features and a target variable, I can create a model.
HVAC: What do you mean by features and target variable?
AI: Pardon me, these are just AI lingo. In the stress example, variables like age, BMI, etc., are called features or independent variables. And the thing you wish to predict—stress level—is called the target or dependent variable.
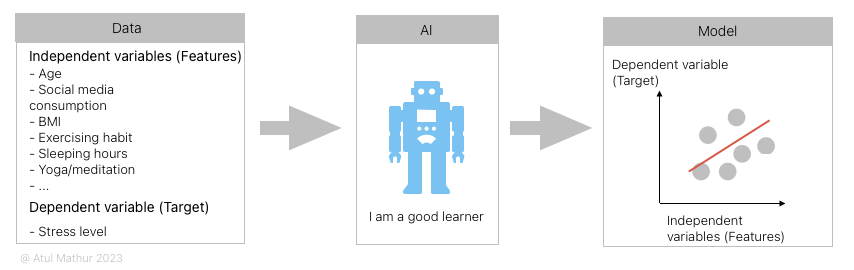
HVAC: So, if I give you a set of features and target values from my side, you can establish a reliable and accurate relationship between them. And then, I can use that model to predict the target values.
AI: Exactly! Let’s talk about your needs.
HVAC: Four buckets and three priorities
HVAC: First, let me give you a glimpse of the stock of your favorite drink: data. Basically, one can pack the HVAC plant’s data into four buckets as shown in the diagram below.
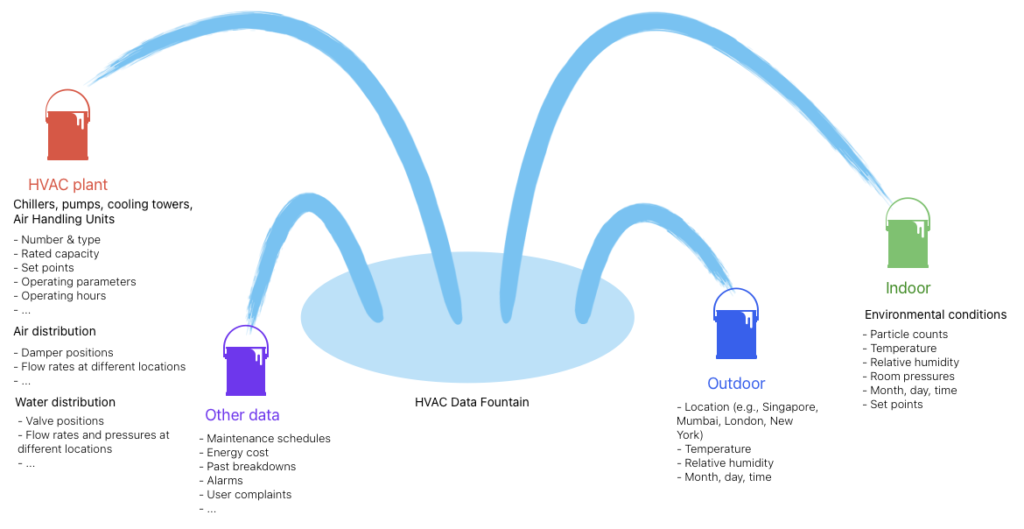
AI: You’ve got a mouthwatering amount of delicious data. So much can be done with it, but my question is simple: What’s your core problem that you would like to solve by involving me?
HVAC: Well, before talking about the problem, let me share my three key priorities:
- Functional performance: I must work hard to provide what users are looking for. For example, in commercial buildings, it’s comfort (temperature, humidity, and air quality). In industrial applications like a pharmaceutical plant, it is cleanliness (particle counts), contamination control (by maintaining pressure cascade between rooms), and of course, precise temperature and humidity.
- Reliability: Besides functional performance, I must not break down at odd times. It can create tremendous inconvenience and monetary loss. I must stay healthy while minimizing maintenance costs.
- Energy: As I mentioned earlier, I enjoy drinking energy, but this drink is getting costlier and has a negative side effect on the environment. So I must cut down on my consumption.
AI: Okay, I got it: For you, the key objectives are functional performance, reliability, and energy saving. So what’s your problem? Most people try to use me without defining a real problem—and that’s not useful at all.
Problem definition
HVAC: Here is how I would like to define my problems but mind it…this is my initial take:
Energy
- For me, energy consumption is an important target or dependent variable. It depends on a number of independent variables or features: plant capacity, working hours, loading, set points, outside weather conditions, indoor conditions, the efficiency of our equipment, etc. Most of the time, we keep running the plant based on fixed set points without much optimization.
- But if someone like you can create a model and then advise me in real time what should be the most optimum operating set points (say, leaving chilled water temperature at chiller or entering water temperature at condenser or AHU return air temperature or fresh air dehumidification set point) that could really help me.
Functional performance
- We have a well-known issue across the HVAC world: Uneven temperatures. In the same office, some people will be overcooling, while others will be feeling warm. In this case, our target variable could be temperature and humidity, while the independent variables could be occupancy, proximity to a window, the density of heat-generating equipment, and supply air temperature and flow rate. Maybe, you could help to optimize the air distribution so that we have uniform temperatures across the facility.
Reliability
- Typically, we follow a fixed schedule for maintenance. But sometimes, we lose track of the health of our equipment and end up with breakdowns. Basically, it is not optimized. If, based on historical data and your modeling, you could advise me when a particular chiller or pump is going to fail, I could schedule maintenance accordingly. Sometimes, we may be overdoing the maintenance, and we could scale it down based on your projections of equipment condition.
AI: Bro, I could really help you on all three fronts. All I need is…data to create a model first. Once I create a model, I can analyze your fresh data in real time and predict and advise you on what to do. In fact, I have the capability to learn from new data continuously, so my model can keep getting refined.
HVAC: Bro, we had a good chat. Before we end, a personal question: Don’t you get sick of consuming so much information and data? I mean, any indigestion problem?
AI: Bro, I love data. I thrive on it. The more I have, the better I feel. But I do get indigestion when people provide me with low-quality data (missing stuff, inaccurate, irrelevant).
HVAC: I understand! Before we try to involve you, we must get hold of our data and ensure its quality. And also define our problem properly.
AI: That’s right! Two things are critical for our future partnership: (1) Pay huge attention to all the data you generate. That’s an asset. (2) Define your problems or challenges as precisely as possible. I would add one more point…start small!
AI: Before we end, I must say thank you. If you don’t cool all these data centers, how would I survive?
HVAC: You’re welcome! Let’s catch up some other day to discuss your two other capabilities: classification and clustering. It was indeed exciting to meet you. I feel younger.
AI: I feel wiser, Bro! Cheers!
Upcoming…
I will be offering a training program titled “HVAC for Biopharma Professionals” later this month. Check it out here.